The Future of Business: Leveraging Video Labeling Tools in Machine Learning
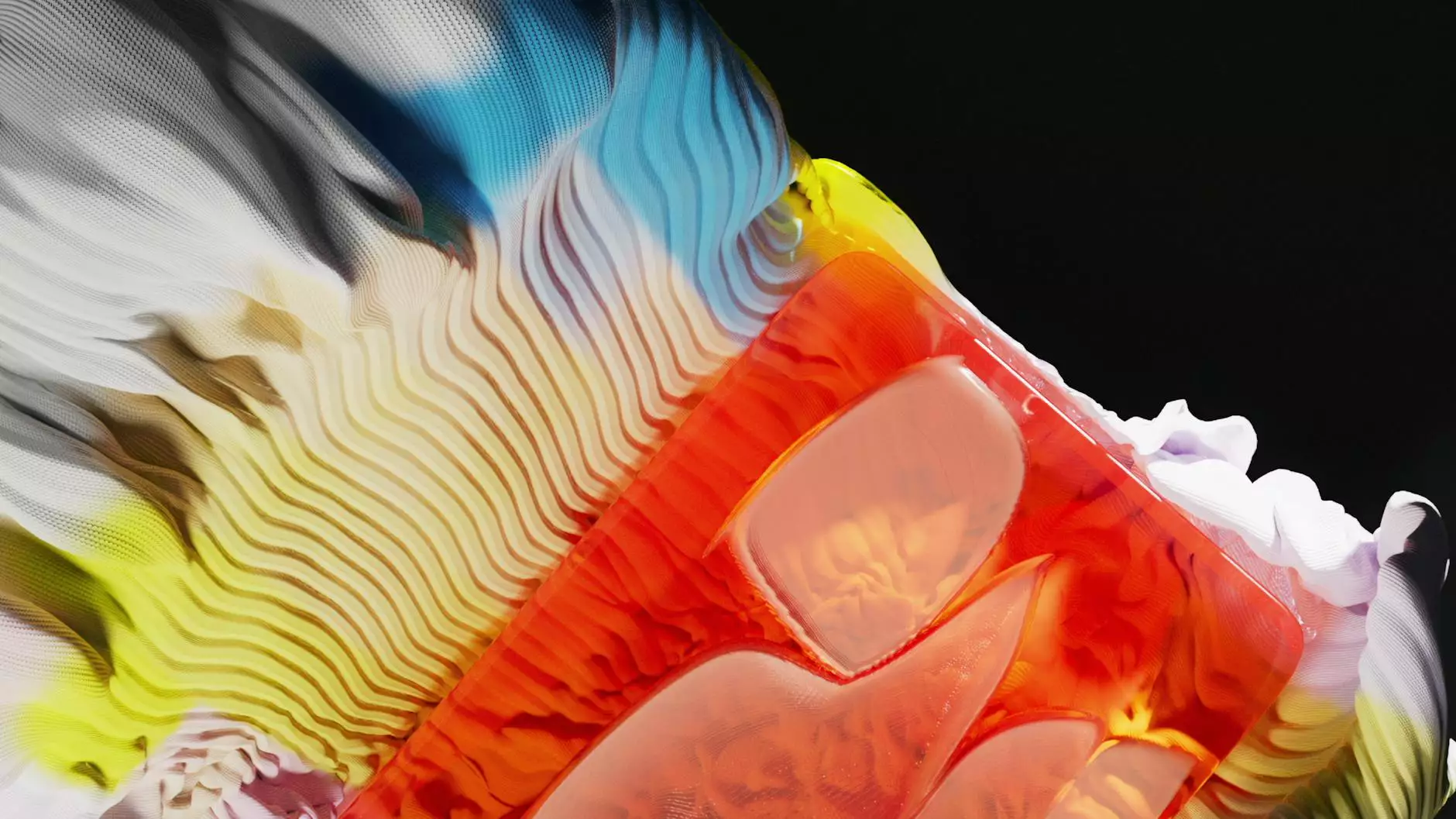
In today's fast-paced technological environment, businesses are increasingly turning to artificial intelligence (AI) and machine learning (ML) to gain a competitive edge. A critical aspect of successful AI implementations involves the use of robust video labeling tools, which facilitate efficient data annotation. This article explores the profound impact of these tools on machine learning initiatives and business productivity.
Understanding Video Labeling Tools
Video labeling tools are specialized software applications designed to annotate videos with metadata, enabling machine learning algorithms to analyze and learn from visual data. These tools are vital in various industries, including healthcare, automotive, entertainment, and security.
What is Data Annotation?
Data annotation is a process where raw data, such as videos, images, or text, is labeled with relevant information. This enriches the data, making it suitable for training ML models. In the case of videos, labeling can involve:
- Object Detection: Identifying and marking specific objects within a video frame.
- Activity Recognition: Annotating actions or behaviors demonstrated in the video.
- Scene Labeling: Classifying scenes or segments based on their content.
The Importance of Video Labeling in Machine Learning
Machine learning models rely heavily on the quality and quantity of input data. The better annotated the data, the more accurately the model can learn and make predictions. Here are a few reasons why video labeling is crucial:
Enhances Model Accuracy
Accurate annotations lead to improved model performance. When ML models are fed well-labeled video data, they can identify patterns, make decisions, and recognize anomalies with significantly better accuracy.
Facilitates Automated Learning
With the integration of powerful video labeling tools, businesses can automate the data annotation process, reducing the time and human effort involved while increasing efficiency.
Supports Real-time Analysis
Organizations can utilize video labeling tools for real-time recognition and analysis, making it easier to respond rapidly to emerging trends or issues within their environments.
Key Features of an Effective Video Labeling Tool
When choosing a video labeling tool for machine learning needs, consider the following features:
1. User-friendly Interface
A tool with an intuitive UI allows teams to get started quickly without extensive training.
2. Compatibility with Various Formats
Ensure the tool supports a wide range of video formats to accommodate different project requirements.
3. Collaborative Annotations
The ability for multiple team members to work on video labeling simultaneously can significantly speed up the data preparation process.
4. Quality Control and Review Mechanisms
Incorporating review processes ensures that the annotations are accurate and consistent across the dataset.
5. Integration with Machine Learning Frameworks
Your chosen tool should easily integrate with popular ML frameworks like TensorFlow and PyTorch for seamless project progression.
Advantages of Using Keylabs.ai
With many tools available, Keylabs.ai stands out in the realm of data annotation platforms focused on video labeling:
1. Advanced Machine Learning Capabilities
Keylabs.ai offers advanced algorithms to automate the labeling process, reducing time spent on manual tasks.
2. Customizable Solutions
Our platform provides customized solutions tailored to meet specific business needs, ensuring compatibility with your operational strategy.
3. Scalability
As your business grows, so can your video labeling initiatives, with options for scaling up without compromising quality.
4. Assistance and Support
Keylabs.ai provides comprehensive support and resources to assist businesses in making the most out of their video labeling tools.
Real-World Applications of Video Labeling Tools
The applications of video labeling tools powered by machine learning are vast. Here are some notable examples:
1. Healthcare
In healthcare, video labeling is used for analyzing patient interactions, monitoring surgical procedures, and enhancing telemedicine diagnostics.
2. Autonomous Vehicles
The automotive industry uses video labeling for recognizing and interpreting the environments around self-driving cars, improving their safety and functionality.
3. Retail Surveillance
Retailers utilize video analytics to assess consumer behavior, enhance security measures, and optimize store layouts.
4. Sports Analytics
Sports teams deploy video labeling to analyze player performance, strategy effectiveness, and refereeing decisions.
Challenges in Video Labeling
Despite the advantages, businesses may face challenges when implementing video labeling tools:
1. Data Privacy and Compliance
Ensuring compliance with data protection regulations is crucial, especially in sensitive sectors like healthcare.
2. Quality Assurance
Maintaining the quality of annotations can be taxing without robust review and control processes.
3. High Initial Investment
Investments in advanced tools can be high upfront, but they often yield significant long-term gains.
Conclusion: Embrace the Future with Keylabs.ai
Investing in a proficient video labeling tool powered by machine learning is not just a technological upgrade; it’s a strategic move that can drive your business towards new heights. As industries continue to evolve, the ability to analyze and derive insights from video data will become increasingly important.
Explore how Keylabs.ai can revolutionize your approach to data annotation and machine learning, offering tailored solutions that fit your unique business needs. By leveraging our advanced video labeling tool machine learning, you can elevate your AI initiatives, turning vast amounts of video data into actionable insights that foster growth and innovation.
Call to Action
Ready to take your video data analysis to the next level? Contact Keylabs.ai today to learn more about how our platform can enhance your machine learning projects. Let's embark on this journey together!